From colleagues to collaborators, a cross-department conversation links statistics to plant science
Complicated sets of biological data can be challenging to extrapolate meaningful information from. Wanting to find a better way to look at this data led Berkley Walker, assistant professor at the MSU-DOE Plant Research Laboratory (PRL) and the Department of Plant Biology, to team up with statistician and Assistant Professor Chih-Li Sung from the Department of Statistics and Probability.
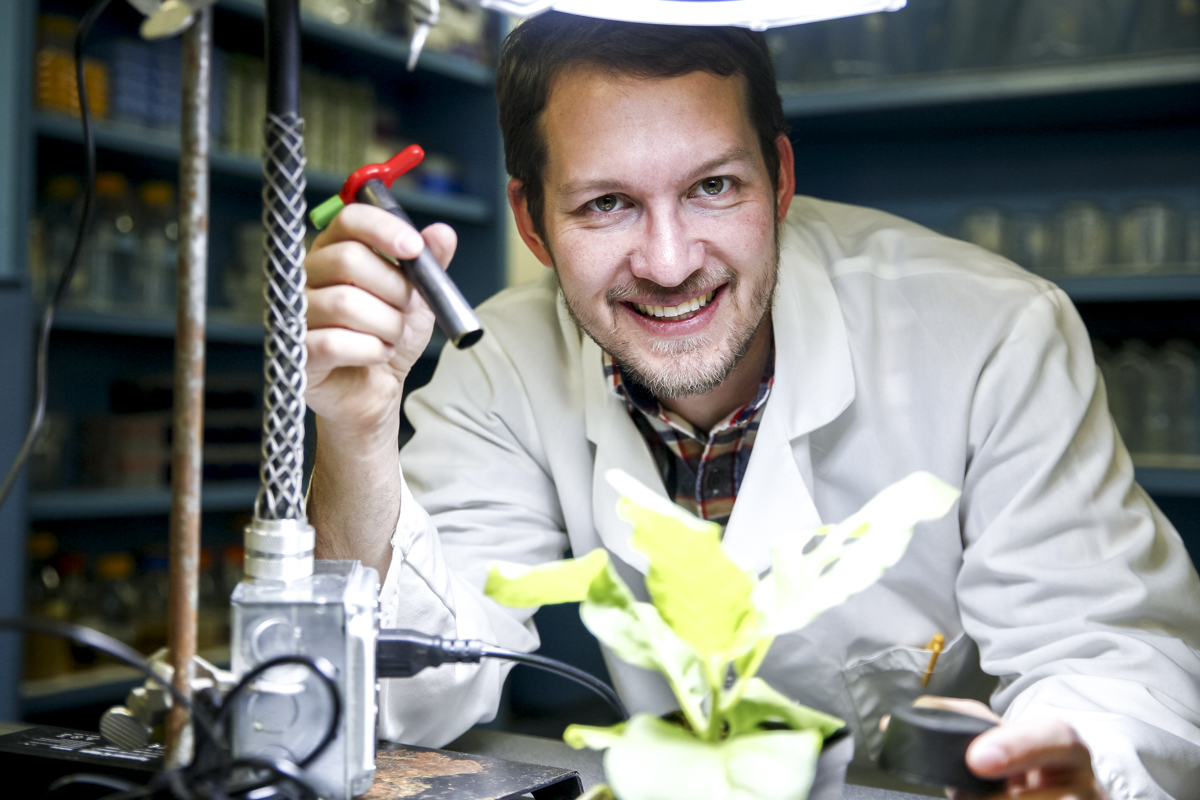
By Chelsea Mamott
The two assistant professors, using data from graduate student Beau David Barber from the University of Illinois at Urbana-Champaign, worked together on finding a new statistical framework that can accurately estimate the parameters in biological models. The findings are published in the SIAM/ASA Journal on Uncertainty Quantification.
Walker and Sung first met at orientation when they joined MSU in 2018. The pair continued their working relationship and eventually developed the idea to work together on this project.
“Interdisciplinary research is important to find what tools that are known in one area that might help in others,” Walker said. “In this case, the ability in statistics to deal with messy data sets can help us better understand our data in ways we wouldn’t be able to come up with ourselves.”
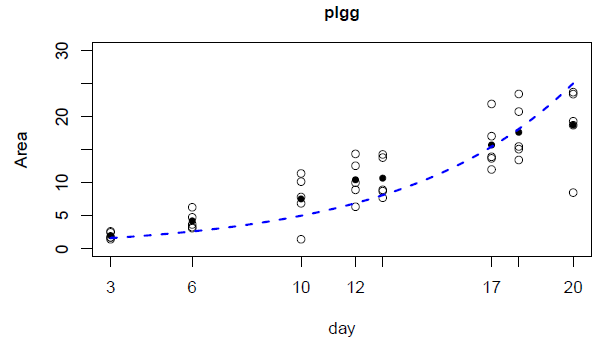
By Chih-Li Sung
Barber collected data on the relative growth rate of Arabidopsis thaliana, a model plant often used in research settings. He recorded information on the size of the plant over time. Then, Sung plotted a line that best fit the growth rate and came up with an equation that fit the line and the data. The basic idea of this resulting “relative growth rate” calculation has been around for a while, but the collaboration with Sung let them better interpret the results statistically.
“Based on the new method, we observe that relative growth rates under ambient CO2 concentrations are slower than under high CO2 concentrations,” said Sung, which was not apparent using statistics usually applied to these types of data sets by plant biologists. “We also observe that [some plants] have slower relative growth rates than wild type plants under ambient CO2 concentrations.”
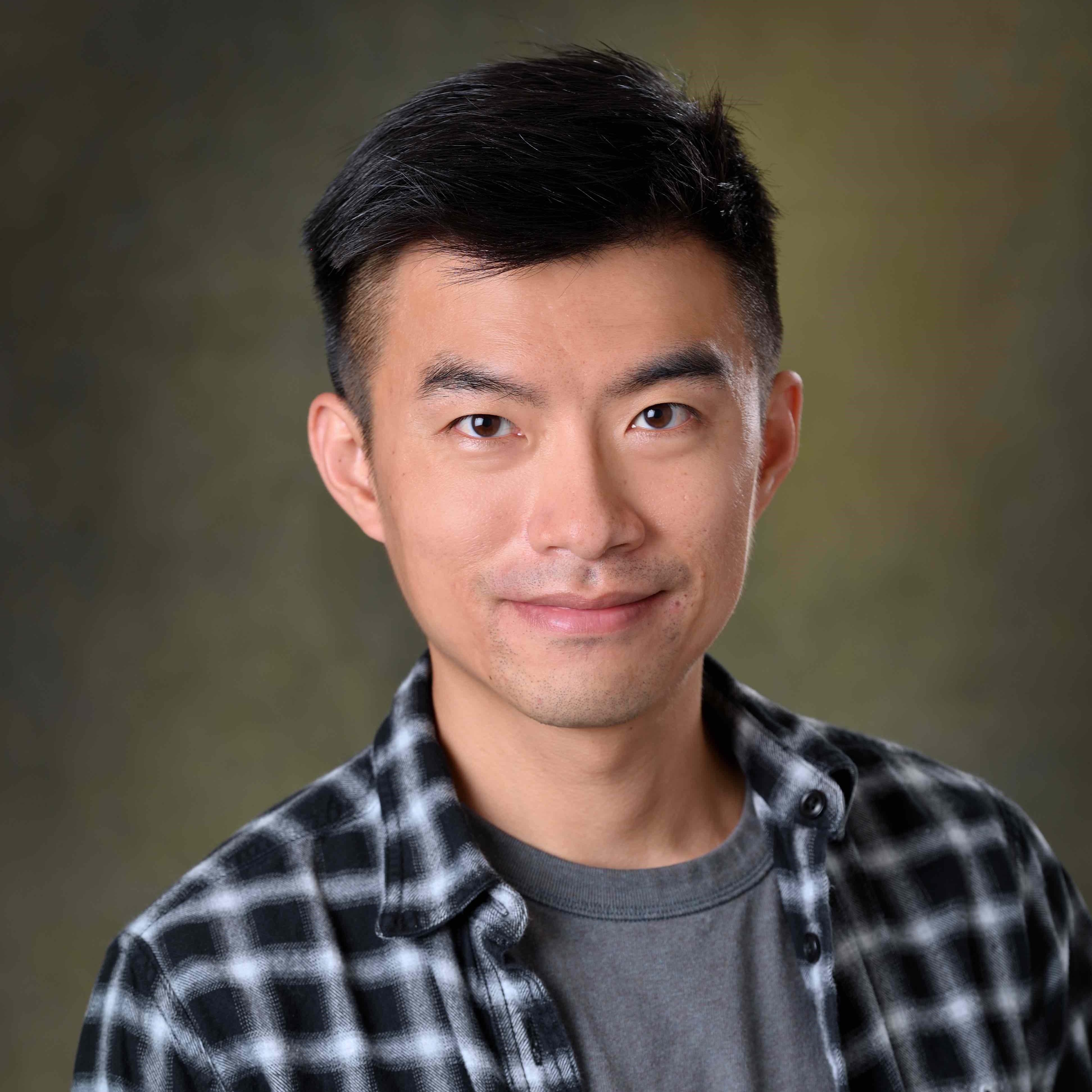
By Harley Seeley
This model can be adapted to fit other messy data sets within plant research, such as in calculating metabolic flux or ecological models. As the work continues, the researchers hope to apply this framework to even more complex data sets.
“It was fun to collaborate with Berkley,” Sung said. “His passion for plant biology has inspired me to devote to computational plant science with the goal to improve biological models with the aid of novel statistical methods.”
Walker added: “It has been a delight to work with Chih-Li, he has been a patient teacher as we have worked together.”
This research was supported by the Division of Chemical Sciences, Geosciences and Biosciences, Office of Basic Energy Sciences of the U.S. Department of Energy Grant DE-FG02-91ER20021.
By Kara Headley